March 29, 2022
How Can Scientists Use Artificial Intelligence (AI) to Improve Predictions of River Water Quality?
Managing water quality in rivers needs a new class of models that are enabled by artificial intelligence and machine learning.
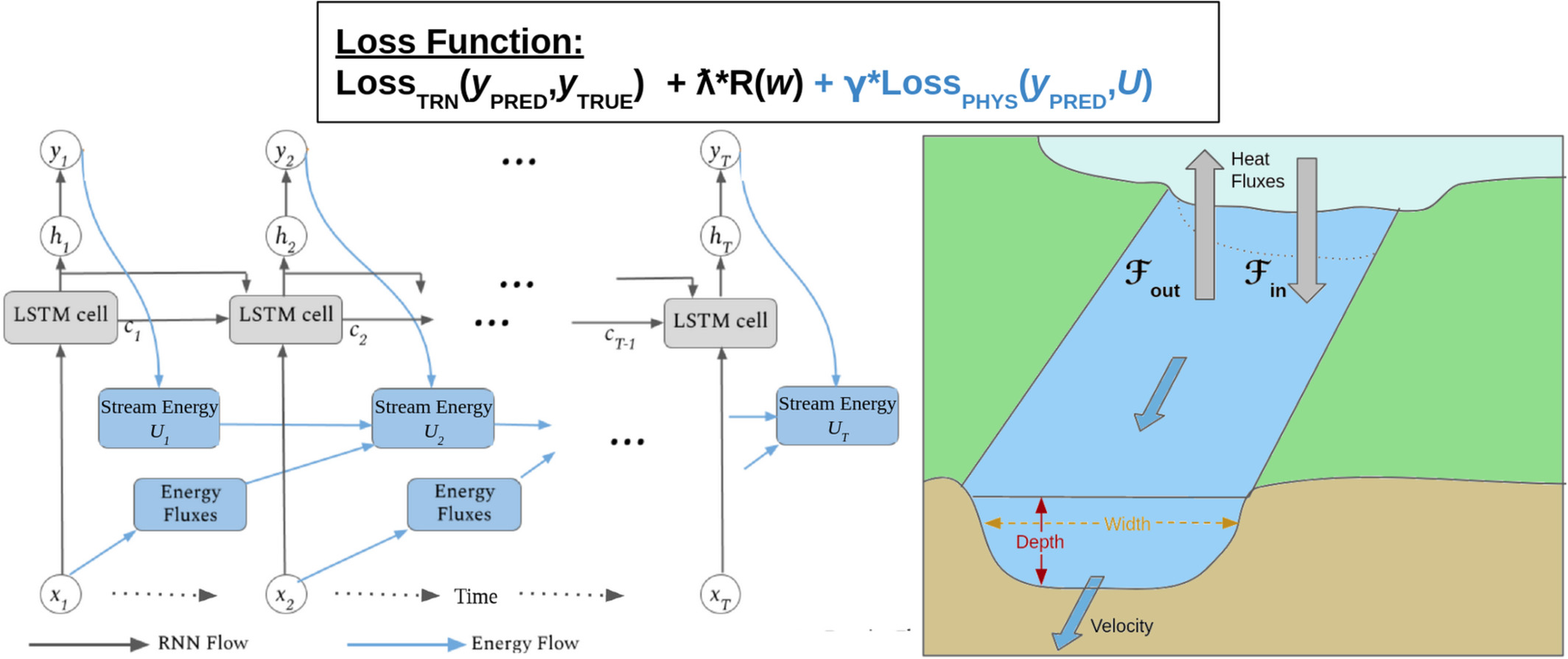
Combining physics-based and machine learning models is potentially a powerful way of enabling more accurate, fast, and trustworthy predictions.
[Reprinted under a Creative Commons Attribution 4.0 International License (CC BY 4.0) from Varadharajan, C., et al. “Can Machine Learning Accelerate Process Understanding and Decision-Relevant Predictions of River Water Quality?” Hydrological Processes 36 (4), e14565 (2022). DOI:10.1002/hyp.14565.]
The Science
Growing populations and climate change are stressing water quality in rivers and streams across the world. Water managers need good models to predict river water quality in rivers. However, current models cannot account for the complex factors that influence water quality. This paper discusses how the latest advances in machine learning and artificial intelligence can improve models of water quality in watersheds and river basins.
The Impact
Better water quality models can help water managers make optimal decisions on water use and treatment. Artificial intelligence (AI) and machine learning methods can enable faster, more accurate predictions of river water quality that are relevant for decision making. However, there are many considerations for how such models should be designed. Watershed managers need predictions that are both accurate and robust to choices for how the model was built. They also need predictions that are explainable and trustworthy to help those who use the models (stakeholders). This study discusses how to design models that are enabled by AI technologies to serve these purposes.
Summary
Watershed managers need to adapt to multiple stressors that include population growth, land use change, global warming, and extreme events. Water managers need good models to predict river water quality in rivers to make optimal decisions on water use and treatment. However, current models cannot account for the complex factors that influence water quality. This paper discusses how artificial intelligence and machine learning can enable more accurate, fast, and scalable models for river water quality. It provides (1) reviews of relevant state-of-the art machine learning applications for water quality and (2) descriptions of opportunities for selecting and designing a new class of models that make use of AI technologies. These models should be designed so that the predictions are accurate and robust to choices for how the model was built. Watershed managers also need predictions that are explainable and trustworthy to help stakeholders who use the information from the models. Successful integration of machine learning into water quality models can help make better water management decisions to plan for an uncertain future.
Principal Investigator
Charuleka Varadharajan
Lawrence Berkeley National Laboratory
[email protected]
Co-Principal Investigator
Eoin Brodie
Lawrence Berkeley National Laboratory
[email protected]
Program Manager
Jennifer Arrigo
U.S. Department of Energy, Biological and Environmental Research (SC-33)
Environmental System Science
[email protected]
Funding
This research was supported by the U.S. Department of Energy’s (DOE) Office of Science program in Biological and Environmental Research (BER).
References
Varadharajan, C., et al. "Can Machine Learning Accelerate Process Understanding and Decision-Relevant Predictions of River Water Quality?." Hydrological Processes 36 (4), e14565 (2022). https://doi.org/10.1002/hyp.14565.