September 13, 2024
Improved Estimation of Carbon Uptake by Arctic and Boreal Ecosystems
Remotely sensed data, machine learning, and improved model parameterization reduced model bias and improved simulated carbon uptake.
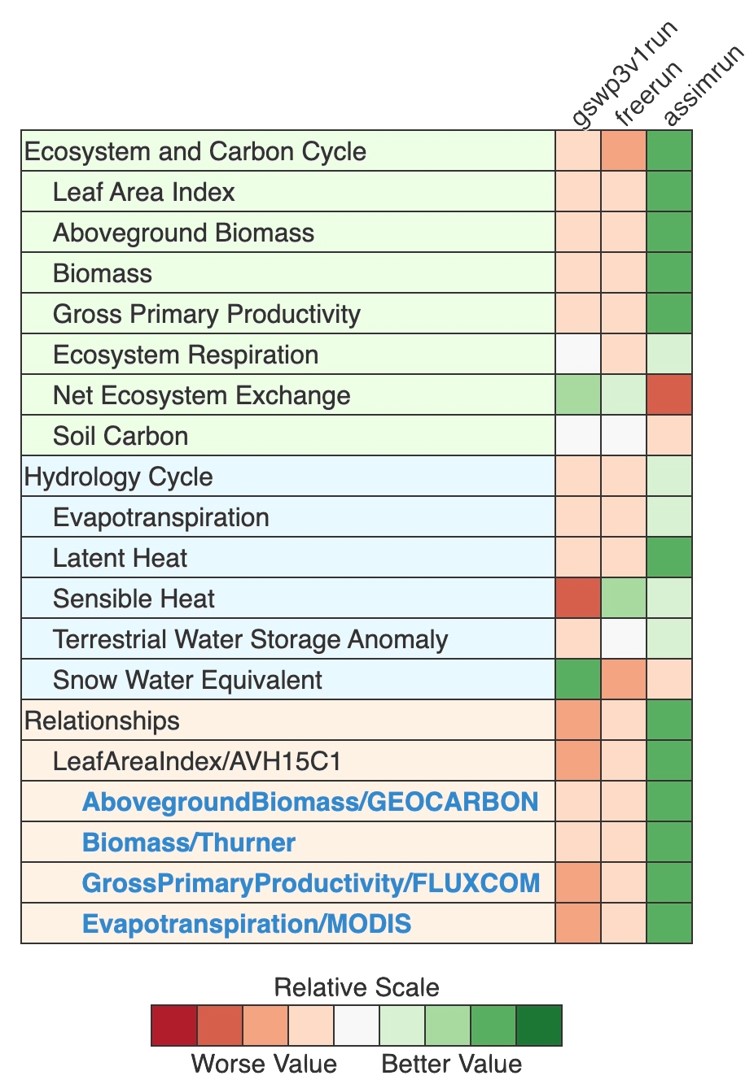
International Land Model Benchmarking Project summary diagram for the default Community Land Model 5 run driven by GSWP3v1 forcing (gswp3v1run), free run, and data assimilation run. The color represents the overall score described in the methodology.
[Reprinted with permission from Huo., X, et al. "Integrating State Data Assimilation and Innovative Model Parameterization Reduces Simulated Carbon Uptake in the Arctic and Boreal Region." Journal of Geophysical Research – Biogeosciences 129 (9), e2024JG008004 (2024). DOI:10.1029/2024JG008004.]
The Science
Arctic and boreal ecosystems are warming rapidly, impacting regional and global carbon cycles. The Community Land Model (CLM) can be used to project future carbon uptake and storage. However, CLM is biased in estimating leaf area index (LAI) and aboveground biomass, which can significantly affect model projections. A team of researchers forced the model estimates of LAI and aboveground biomass to be consistent with satellite-derived LAI observations and a machine learning product of aboveground biomass. Furthermore, the team enabled two key parameters in photosynthesis to vary with leaf temperature. Assessment using the International Land Model Benchmarking Project (ILAMB) showed significant improvement of model projections.
The Impact
Assimilating LAI and aboveground biomass observations into a land model reduced model bias associated with estimating key model outputs. Data assimilation significantly improved the model’s performance in carbon and hydrological cycles, as well as functional relationships. Implementation of a new parameterization accounting for low temperature sensitivity of photosynthesis further reduced model bias in estimating gross primary productivity (GPP).
Summary
Model representation of carbon uptake and storage is essential for accurately projecting the arctic-boreal zone’s reponse to a rapidly changing climate. Land model estimates of LAI and aboveground biomass that can have a marked influence on model projections of carbon uptake and storage vary substantially in the arctic and boreal zone, making it challenging to correctly evaluate model estimates of GPP.
To understand and correct bias of LAI and aboveground biomass in CLM, a team of researchers assimilated the 8-day Moderate Resolution Imaging Spectroradiometer (MODIS) LAI observation and a machine learning product of annual aboveground biomass into CLM. The team used an Ensemble Adjustment Kalman Filter in an experimental region including Alaska and western Canada. Assimilating LAI and aboveground biomass reduced these model estimates by 58% and 72%, respectively. The change of aboveground biomass was consistent with independent estimates of canopy top height at both regional and site levels.
The ILAMB assessment showed data assimilation significantly improved CLM’s performance in simulating carbon and hydrological cycles and in representing functional relationships between LAI and other variables. To further reduce the remaining bias in GPP after LAI bias correction, the team re-parameterized CLM to account for low temperature suppression of photosynthesis. The LAI bias–corrected model that included the new parameterization showed the best agreement with model benchmarks. Combining data assimilation with model parameterization provides a useful framework to assess photosynthetic processes in land surface models.
Principal Investigator
David Moore
University of Arizona
davidjpmoore@arizona.edu
Program Manager
Daniel Stover
U.S. Department of Energy, Biological and Environmental Research (SC-33)
Environmental System Science
daniel.stover@science.doe.gov
Funding
Funding was received from NASA Terrestrial Ecology Grant 80NSSC19M0103. High-performance computing support was received from Cheyenne and provided by the Computational and Information Systems Laboratory at the National Science Foundation’s National Center for Atmospheric Research. The study was also supported by the Next‐Generation Ecosystem Experiments (NGEE Arctic) project, which is supported by the Biological and Environmental Research program in the U.S. Department of Energy’s (DOE) Office of Science, and through DOE’s contracts No. DE‐SC0012704 to Brookhaven National Laboratory and No. DE‐AC02‐05CH11231 to Lawrence Berkeley National Laboratory.
References
Huo., X, et al. "Integrating State Data Assimilation and Innovative Model Parameterization Reduces Simulated Carbon Uptake in the Arctic and Boreal Region." Journal of Geophysical Research - Biogeosciences 129 (9), e2024JG008004 (2024). https://doi.org/10.1029/2024JG008004.