March 24, 2022
Regional Stream Temperature Predictions Using Classical Machine Learning Models
New models predict monthly stream temperatures in pristine and human-impacted watersheds.
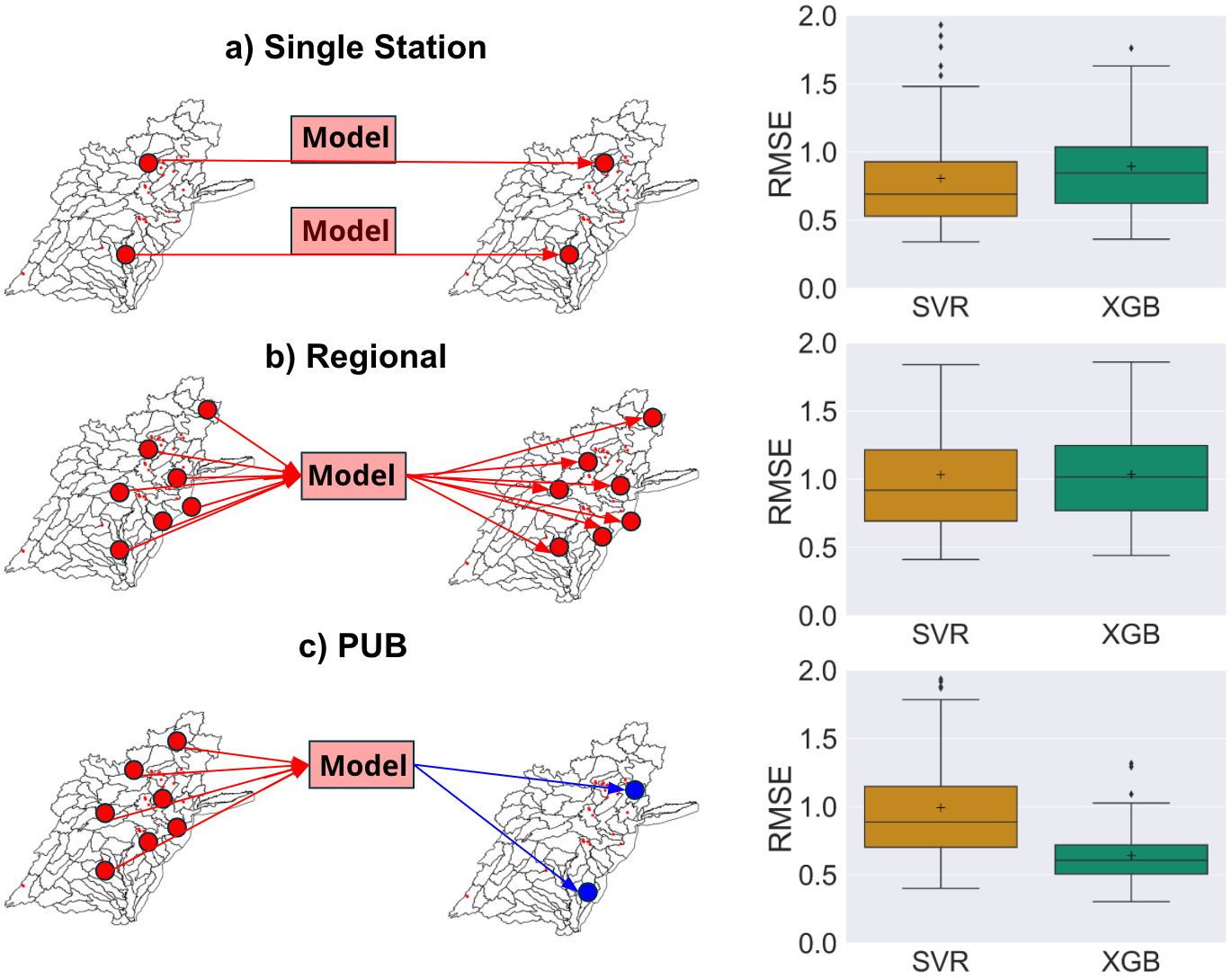
Machine Learning models (SVR, XGB) accurately predicted monthly stream temperatures for 3 scenarios—local (single station, a), regional (b), and for unmonitored basins (PUB, c). Prediction accuracy (RMSE) is shown in degree Celsius.
[Reprinted under a Creative Commons Attribution 4.0 International License (CC BY 4.0) from Weierbach, H., et al. “Stream Temperature Predictions for River Basin Management in the Pacific Northwest and Mid-Atlantic Regions Using Machine Learning.” Water 14 (7), 1032 (2022). DOI:10.3390/w14071032.]
The Science
Researchers used classical machine learning models to predict monthly stream temperatures for 78 pristine and human-impacted watersheds in the Mid-Atlantic and Pacific Northwest hydrologic regions with diverse geologies, climate, and land use. The models improved local and regional prediction accuracies by 15 to 48% relative to a baseline statistical model. Results showed that air temperature was the primary factor affecting monthly stream temperature, indicating that the models could be used with a minimal amount of input data on climate variables that are broadly available. These models enable predictions of stream temperature at new sites, such as unmonitored and dammed watersheds.
The Impact
Stream water temperature is a master water quality variable expected to increase in the future due to climate change. Water managers need accurate stream temperature predictions to make optimal decisions. Researchers found that machine learning could be used to accurately predict monthly stream temperatures, both locally and regionally, in pristine and human-impacted watersheds. Such monthly models can play an important role in near-term seasonal forecasting to plan for and understand future impacts on stream temperatures due to a changing climate and extreme events.
Summary
Stream water temperature is an important water quality variable that affects river ecosystem health and water use. Short and long-term predictions of stream temperatures are needed to make optimal water management decisions that account for a changing climate and extreme events. This study used classical machine learning models (support vector regression, gradient boosted trees) to predict monthly stream temperatures in 78 watersheds of the Pacific Northwest and Mid-Atlantic regions in the United States, which have diverse climate, land use and geologies. The models used input data on climate and stream flow records with basic watershed information (location, elevation, size). The models were used for local, regional, and unmonitored scenarios and improved prediction accuracies by 15 to 48% relative to a baseline statistical model. Results showed that air temperature was the primary factor affecting monthly stream temperature, indicating that the models could be used with a minimal amount of input data on broadly available climate variables. These results will expand the capabilities of models to predict stream temperature at new sites—such as in watersheds with dams, and for watersheds that lack extensive historical data or other information describing their properties (e.g., extent of land cover, number of dams).
Principal Investigator
Charuleka Varadharajan
Lawrence Berkeley National Laboratory
cvaradharajan@lbl.gov
Funding
This research was supported by the U.S. Department of Energy’s (DOE) Office of Science Biological and Environmental Research (BER) Program.
Related Links
References
Weierbach, H., et al. "Stream Temperature Predictions for River Basin Management in the Pacific Northwest and Mid-Atlantic Regions Using Machine Learning." Water 14 (7), 1032 (2022). https://doi.org/10.3390/w14071032.