February 01, 2022
New Workflow Unifies Community-Wide Adsorption Data, Unlocking New Modeling Capabilities
Publicly released work package enables comprehensive modeling of adsorption reaction constants and unlocks artificial intelligence-machine learning applications.
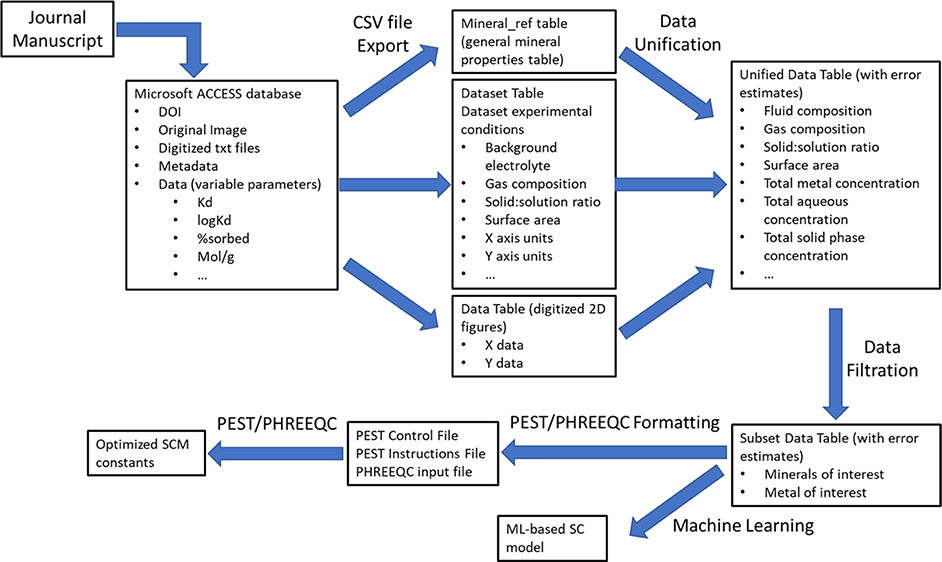
L-SCIE workflow to enable comprehensive modeling of surface complexation reaction constants.
[Reprinted under a Creative Commons Attribution 4.0 International License (CC BY 4.0) from Zavarin, M., et al. “Community Data Mining Approach for Surface Complexation Database Development.” Environmental Science & Technology 56(4) 2827–2838, (2022). DOI: 10.1021/acs.est.1c07109]
The Science
Reactions at the solid-water interface, known broadly as sorption, play an important role in nutrient availability and contaminant transport in the environment. While the field of sorption is rapidly growing, few efforts have been made to capitalize on this rich data source. Because various surface complexation models that describe sorption processes carry differing fundamental assumptions, a present-day challenge exists in unifying the plethora of experimental results published over past decades. The Lawrence Livermore National Laboratory Surface Complexation-Ion Exchange (L-SCIE) database presented in this study demonstrates a path forward by compiling community-based experimental sorption data and conducting a series of transformations to unify, filter, and format the data. The outputted .csv dataset of commonly formatted experimental sorption data enables the application of powerful new modeling techniques, such as artificial intelligence and machine learning methods.
The Impact
This project outlines a comprehensive data analytics workflow to mine community-wide sorption data from the literature. Through the development of a consistently formatted data compilation approach, this work enables traditional surface complexation model development and sets the stage for novel artificial intelligence and machine learning algorithms to process large, community-based sorption data for more flexible and accurate modeling predictions.
Summary
This study presents a data-to-model workflow that unifies individual sorption datasets across the research community into a consistently formatted database. Additionally, this project demonstrates the use of a data fitting workflow to efficiently optimize the newly formatted dataset of adsorption reactions. The discussed modeling framework, which performs data digitization and unification, is shown to effectively characterize uranium sorption onto the mineral quartz. The uranium-quartz reaction constants determined from this project captured all sorption data available from the literature. Ultimately, the L-SCIE sorption database presented in this study allows for data pre-processing automation across a wide range of metals and minerals, unlocking an important step towards the implementation of novel machine learning methods in sorption research.
Principal Investigator
Mavrik Zavarin
Lawrence Berkeley National Laboratory
[email protected]
Program Manager
Jennifer Arrigo
U.S. Department of Energy, Biological and Environmental Research (SC-33)
Environmental System Science
[email protected]
Paul Bayer
U.S. Department of Energy, Biological and Environmental Research (SC-33)
Environmental System Science
[email protected]
Funding
This work was supported in part by the U.S. Department of Energy’s Earth and Environmental Systems Sciences Division (EESSD) of the Office of Science Biological and Environmental Research Program under contract SCW1053. Additional support was provided by the Spent Fuel and Waste Science and Technology campaign of the Department of Energy’s Nuclear Energy Program. The University Engagement program of LLNL’s University Relations office also provided funds under contract DE-AC52-07NA27344 to LLNL and DE-AC02-05CH11231 to LBNL.
Related Links
References
Zavarin, M., et al. "Community Data Mining Approach for Surface Complexation Database Development." Environmental Science & Technology 56 (4), 2827–2838 (2022). https://doi.org/10.1021/acs.est.1c07109.